2024.02.28
Bayesian non-parametric inference for the ETAS model and method developments
SOKENDAI研究派遣プログラム 採択年度: 2023
Yuanyuan Niu
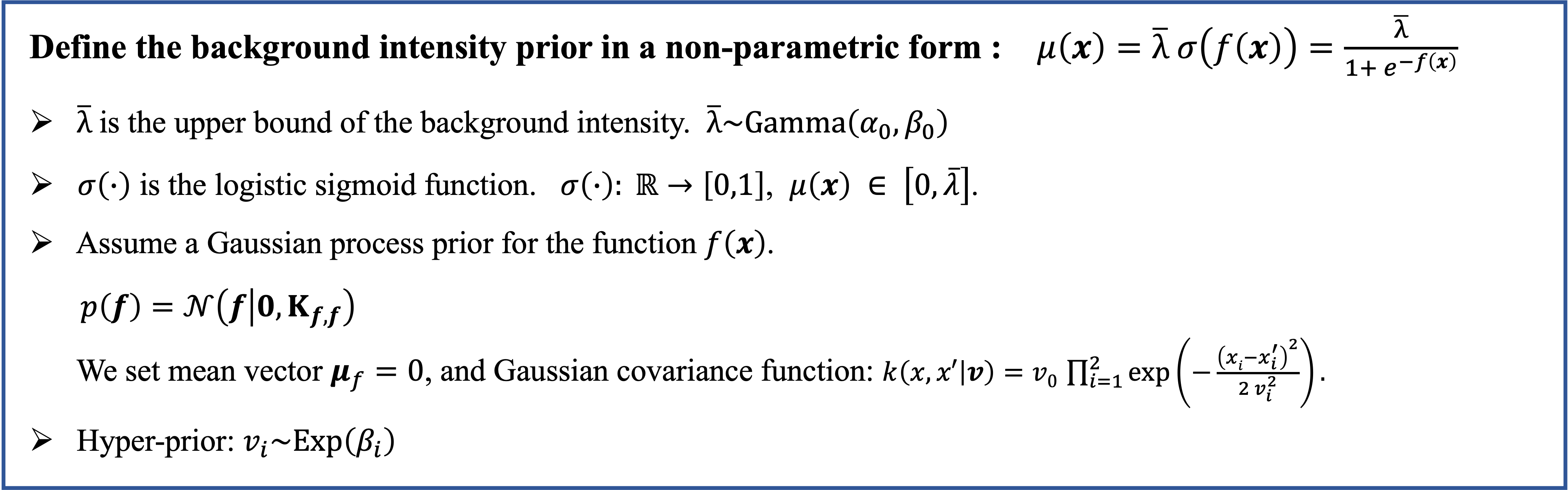
The background intensity is modeled in a Bayesian non-parametric way via a Gaussian Process, which allows us to perform Bayesian inference via Monte Carlo sampling.
Statistical seismology is the application of statistical methods to earthquake science, which is used to help us improve our knowledge of how the earth works. Spatio-temporal point process models are often used in statistical seismology for describing the occurrence of earthquakes (point data). The most widely used one is the epidemic-type aftershock sequence (ETAS) model. Statistical inference of the parameters of the ETAS model is useful to improve our scientific understanding of the mechanism of earthquakes.
In contrast to the traditional statistical inference for the ETAS model, the Bayesian inference allows to incorporate prior information of the parameters and gives a posterior distribution which provides the uncertainty quantification of the parameters. However, many more complex mathematical problems need to be solved in Bayesian inference for the ETAS model. The GP-ETAS model not only solves Bayesian statistical inference problems from a non-parametric perspective but also provides powerful mathematical skills for solving a series of challenging mathematical problems.
GP-ETAS model firstly assumes a Gaussian process (GP) prior for the background intensity in the ETAS model. Secondly, the likelihood representation is rewritten to be conditional conjugate to the priors after introducing auxiliary variables: a latent branching structure, a latent Poisson process, and latent Pólya–Gamma random variables. These three augmentations allow us to implement a Gibbs sampling procedure or mean-field variational inference procedure to obtain the posterior distribution in a Bayesian non-parametric way.
The research group at the University of Potsdam has well completed the Gibbs sampling algorithm, which obtains estimates of parameters by randomly sampling from their conditional posterior. Gibbs sampling is a tractable and efficient method, but its computational complexity is extremely large and time-consuming. Supported by the SOKENDAI dispatch program, I visited this group and discussed some details. We plan to develop efficient tools for computational inference. In addition, some good ideas from GP-ETAS research have inspired me in the study of replenishing missing data in the spatio-temporal Hawkes process. My further research will focus on combining the Bayesian non-parametric inference with the biscale empirical transformation method to solve the missing data replenishment problems.
派遣先滞在期間
Date of Departure: 2023/9/8
Date of Return: 2023/12/04
国、都市等
Potsdam, Germany
機関名、受入先、会議名等
Institute of Mathematics, University of Potsdam
派遣中に学んだことや得られたもの
During my visit, I really enjoyed participating in the weekly meetings with the collaborators who are knowledgeable, responsible, and hospitable people. Their guidance on my research not only facilitated my doctoral research, but also helped me broaden my horizons when thinking about my long-term research topics. I also would like to express my gratitude to the other principal investigators and PhD students of the Data Assimilation project team for providing many communication opportunities, which have left a deep impression on me.
複合科学研究科 統計科学専攻 Yuanyuan Niu
.