2021.10.20
Using Deep Neural Networks for Increasing Speech Intelligibility in Noisy Environment
SOKENDAI Publication Grant for Research Papers program year: 2021
Informatics Li Haoyu
情報学コース
Multi-Metric Optimization Using Generative Adversarial Networks for Near-End Speech Intelligibility Enhancement
journal: IEEE/ACM Transactions on Audio, Speech, and Language Processing publish year: 2021
DOI: https://doi.org/10.1109/TASLP.2021.3111566
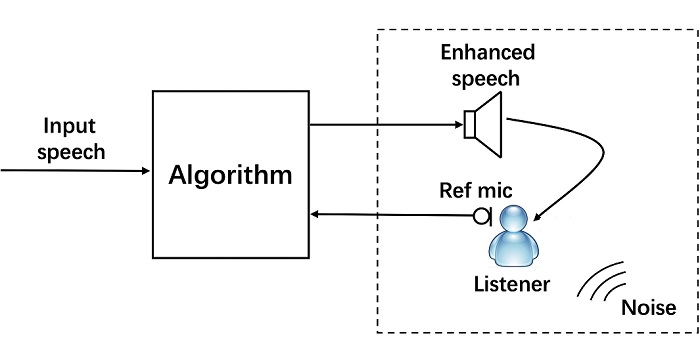
We proposed a new method to improve the speech intelligibility Real-life speech communication, such as mobile telephony and public-address announcement, usually occurs in noisy environments (as shown in dotted square), which results in stressful listening and/or non-understanding for listeners. Our objective is to develop an algorithm to modify the speech signal in such a way as to improve speech intelligibility.
Deep neural network (DNN) is the system comprising of multiple layers of computational nodes to derive high-level function from input information. In this work, by utilizing DNN, our method automatically modifies speech signal to significantly improve its intelligibility while still maintaining the volume of speech. To achieve it, we directly used DNN to assist in optimizing speech metrics. Benefitting from this idea, we found that the intelligibility of speech can be greatly increased. Compared to traditional methods which rely on complicated signal processing scheme, our method is more efficient.
This technique can be used for many practical applications such as announcement in noisy railway station. In the future, we plan to further simplify our model to make it easier to be implemented in devices such as mobile phone and hearing aids.
Bibliographic information of awarded paper
- Title: Multi-Metric Optimization Using Generative Adversarial Networks for Near-End Speech Intelligibility Enhancement
- Authors: Li Haoyu, Yamagishi Junichi
- Journal Title: IEEE/ACM Transactions on Audio, Speech, and Language Processing
- Publication Year: 2021
- DOI: https://doi.org/10.1109/TASLP.2021.3111566
Department of Informatics Li Haoyu